Statistical consultation is especially valuable during the planning stage of a research study or a proposal for extramural funding. The early and continuing participation of a biostatistician always improves a grant proposal and the ensuing research. Interim analyses are valuable elements of progress reports, and aid investigators in adjusting the course of their research. The collaboration of biostatisticians in the final analyses, resulting in abstracts for presentation and publications, greatly strengthens the credibility of the study findings to the cancer research community.
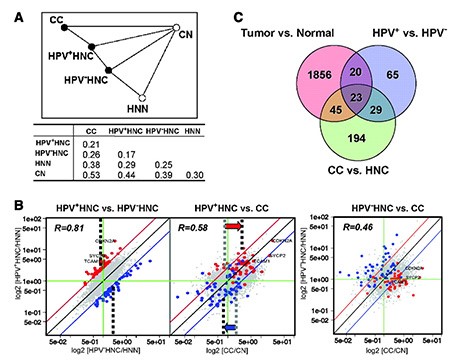
Basic Science
Biostatistician: Michael A. Newton, PhD – Cancer Genetics Program
Collaborators: Paul Ahlquist, PhD and Paul F. Lambert, PhD – Human Cancer Virology Program
I/IIa clinical trial in 22 stage D0 prostate cancer patients was conducted to evaluate the safety of a DNA-based vaccine encoding Prostatic Acid Phosphatase (PAP).
Working with Drs. Lambert and Ahlquist, Dr. Newton analyzed whole-genome profiles from human tissue samples. Findings provided novel biomarkers for early detection and emphasized the potential value of targeting E6 and E7 function in the treatment of HPV+ cancers.
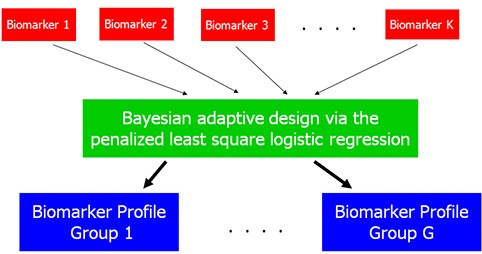
Clinical Science
Biostatisticians: Jens C. Eickhoff, PhD – Experimental Therapeutics Program; KyungMann Kim, PhD – Chemoprevention Program
Collaborators: Jill M. Kolesar, PharmD – Experimental Therapeutics Program; Howard H. Bailey, MD – Chemoprevention Program
Motivated by their collaboration with Drs. Kolesar and Bailey, Drs. Kim and Eickhoff developed optimal clinical trial designs for pharmacogenomics-driven targeted therapies that directly integrated information about biomarkers and clinical outcomes as they become available. The design efficiently identified patients who benefit most from a targeted therapy. There were substantial savings in the sample size requirements when compared to alternative designs.
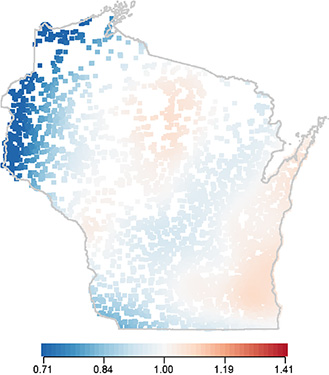
Population-Based Science
Biostatistician: Ronald E. Gangnon, PhD – Cancer Control Program
Collaborators: Amy Trentham-Dietz, PhD, Jane McElroy, PhD, Patrick Remington, PhD, and Polly A. Newcomb, PhD – Cancer Control Program
Using geocoded residential locations for case-control study participants, Dr. Gangnon utilized a generalized additive logistic regression model to estimate geographic risk as a local odds ratio using a two-dimensional thin plate spline while adjusting for established risk factors. Results suggest that established breast cancer risk factors do not explain long-standing observations of higher breast cancer mortality in eastern WI counties.